Monkeying around with Fantasy Baseball Projections
By Clave Jones
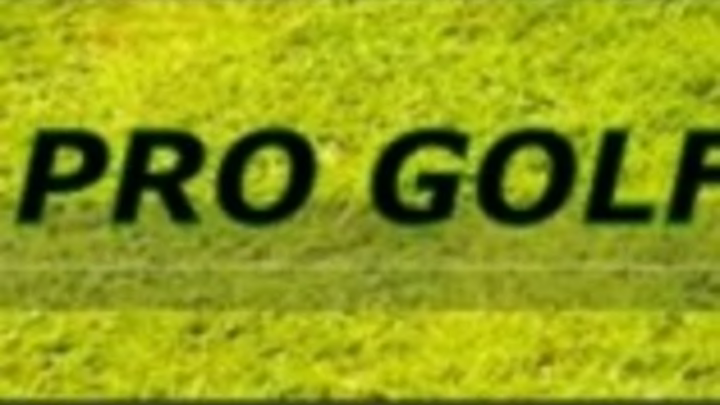
I’ve written before about how we do our fantasy baseball projections. Being that I’m neck-deep in projections for the 2013 fantasy baseball season, I thought I’d share a few lot more thoughts on our methodology.
At the core of our projections is the Marcel system, which was developed by Tom Tango, who in my opinion has done as much for the fantasy baseball community as anyone, including Bill James or Daniel Okrent. Marcels is shorthand for “Marcels the Monkey“, a nod that it’s a fantasy baseball projection method that is so simple a monkey could do it.
There are a dozen or more baseball projection systems out there, many offered for free, many behind a paywall, and one (PECOTA) made famous because Nate Silver, the genius behind it, took his skills to the presidential election. Marcels has stood strong against them all, yet it is far simpler than it’s more complicated and proprietary prognosticating peers.
Crackerjacks uses a modified version of Marcels and I’m going to walk you through a step-by-step of how we do it. The steps are weighting, regression, and aging. We add a 4th, which is conditions.
Weighting
Some systems use comparables as their foundation, but most use multiple years of back data whenever possible. Marcels adds weights to the data, which makes sense because it allows you to place more emphasis on the player’s most recent season, yet still take account for possible random variation.
For example, let’s consider a player who has a home run per plate appearance of .037 in his most recent season, .047 the year before, and .053 the year before that. Simply averaging these gives us a .046.
But in order to look toward the years ahead it’s becomes more accurate to place more weight on the most recent season and Marcels does that. The weights are 5/4/3, with 5 going to the most recent season.
You multiply the component figure by the weights, add them, then divide the total by the weight total. Continuing our example, the weighted sum would be (.037*5) + (.047*4) + (.053*3) = .532. The sum of the weights is 5+4+3 = 12, making our weighted average .532/12 = .044.
Multiply this figure with your projected number of plate appearances and there is your number for home runs hit. .044 X 575 PA = 25.3 HRs.
Our example was Carlos Gonzalez. You might remember that Cargo had a breakout in 2010 and hit 34 homers, but had just 22 last season. We’ve given each season a weight so you might assume that we’re saying Cargo will hit 25.3 home runs next season, but not so fast. We still have 3 more steps.
Regression
Regression to the mean can be a misunderstood thing, so let’s start with a list of the top ten players last season. This a list of great players who had a great season, but next year’s list won’t see all 10 players take a step forward. In fact, you’ll see a slight decrease in the numbers form the majority of the players. This is why projection systems regress to the mean, getting a better estimate of the player’s true talent level, stripping out factors such as luck or randomness or an expectation to improve on a fantastic season.
Most forecasting systems get pretty complicated here. Some regress each position their their own mean. Others find comparable players as a population, or use age, skill-type, etc., but Marcels simply lumps the entire pool together. This is true for components as well. Some skills stabilize more quickly, such as strikeout rate, while others take more plate appearances. Related, aplayer with fewer major league plate appearances may need to regress more because we know less about him and his skills. Again, Marcels lumps the entire pool together.
Our example, Cargo, had 636 plate appearances in 2010, 542 in 2011, and 579 in 2012. Multiply those by the appropriate weights (636*3+542*4+579*5) and you get 6,971, which we’ll use to get his individual reliability score. Here’s the formulation: r=PA/(PA+1200) or 6971/(6971+1200) =.85
So Cargo’s reliability is .85 or (1-.87) = 15%. I must admit that I’m not entirely sure where Tom Tango got his 1200 figure, but Marcel defines reliability as the sum of the weighted plate appearances divided by itself, plus 1200.
Why am I killing you with math and words like ‘regression’, you ask? Well, beginning to think about a reliability (r) score for players is very helpful in evaluating fantasy baseball players. Many fantasy baseball owners had their first place hopes dashed by Brett Lawrie’s mediocre performance and Eric Hosmer’s poor performance. Lawrie had just 171 major league plate appearances for a reliability score of just .12 and Hosmer 563 for a r of only .32 (higher is better, like .75+ or more). Obviously, we have minor league numbers and scouting reports, so I’m not saying their projections were completely unwarranted. I’m just saying that we didn’t have enough data to say they had a high level of reliability, meaning you need to draft with that in mind. Get a mix of high reliability players to form you fantasy baseball team’s foundation, then sprinkle a few “low-r” guys in there.
If we know a player’s reliability, it’s easy to find the regressed rate. It is:
"Regressed rate = (Player r * Player Rate) +((1 – Player r) * League Rate)"
I feel like I’m losing you (maybe I’ve lost it myself), so let’s cut straight to our example and keep moving. We’ve found Cargo’s reliability to be .85, and earlier found his home run rate to be .044, against a league average that is .026 (thank you Baseball Reference). Easy: (.85*.044) + (.15*.026) = .041, meaning Cargo’s regressed home run rate is .041.
First, don’t just average 3 years of data. Second, our brains can see false patterns. If a player hits 10 in year one and 15 the next, we jump to the conclusion that he’s sure to hit 20 in year 3. Either way, it’s better to regress to the mean using the reliability rate as a guide. Look at this as one part player and one part population. The more we know of a player the more we lean on him. The less we know about a player the more we lean on the general population of players.
Aging
Finally, Marcels takes into account an age adjustment. As players age, they either see improvement or decline. Although each component skill has a different aging factor (power peaks before walk rate, for example), in general, players see a improvement in their performance until about age 27-28, then see a steady decline afterwards.
Age | Multiplier |
20 | 1.054 |
21 | 1.048 |
22 | 1.042 |
23 | 1.036 |
24 | 1.030 |
25 | 1.024 |
26 | 1.018 |
27 | 1.012 |
28 | 1.006 |
29 | 1.000 |
30 | 0.997 |
31 | 0.994 |
32 | 0.991 |
33 | 0.988 |
34 | 0.985 |
35 | 0.982 |
36 | 0.979 |
37 | 0.976 |
38 | 0.973 |
39 | 0.970 |
40 | 0.967 |
Age 29 doesn’t get a multiplier because that year would be looking at the age 26-28 seasons, which is right in the middle of his talent level.
With Cargo, his age-adjustment rate gives his home run rate a gentle adjustment, being that Cargo is right in the think of the curve skill-wise. Regressed rate (.0413) X age adjustment (1.012) = .042, which can be simply multiplied by his estimated plate appearances (575) and you have yourself a projected home run total of 24. Set up your spreadsheet to do this for each component stat and you’re up and running.
The Marcel system factors just three things, yet consistently is shown to be hang tight with much more complex projection systems.
Conditions
Crackerjacks adds one more step, which we don’t finalize until the very last hour. We use our eyes, experience, and gut, thinking about the conditions in which the player finds themselves.
You learn quickly to understand the “he’s in the best shape of his life” statements for what they are, but where a player bats in the order, a change of stadium, nagging injuries, off-the-field issues, etc. really matter. Some systems to their credit factor in as many of these variables as they can, but don’t adjust the numbers once the computers spit them out.
Marcels gives a great mathematical foundation for component skills, but estimating plate appearances in particular is still more of an art than a science. If a number jumps out at us (maybe it’s a spreadsheet error, a player with an unusual skillset, or it doesn’t fit with what we are hearing from Spring Training) we give consideration the fact that are humans playing a game and we’ll tick it up or down, adjusting only slightly form our mathematical foundation, but adjusting all the same.
The above is a lot of numbers to digest and my fear, of course, is that I’ve failed in explaining this clearly and only added to the confusion. Worse yet, I fear that Tom Tango himself will comment that I’ve forgotten to carry my aught. Greater than those fears, however, is my belief that’s it’s good to have transparency about process. I wanted to let you behind the curtain so that you’d feel confident and enthused when you use our projections to prepare for your future fantasy baseball teams. We work hard on them and we use them for our own fantasy baseball teams, and we hope that you find them valuable in building your own fantasy baseball teams.